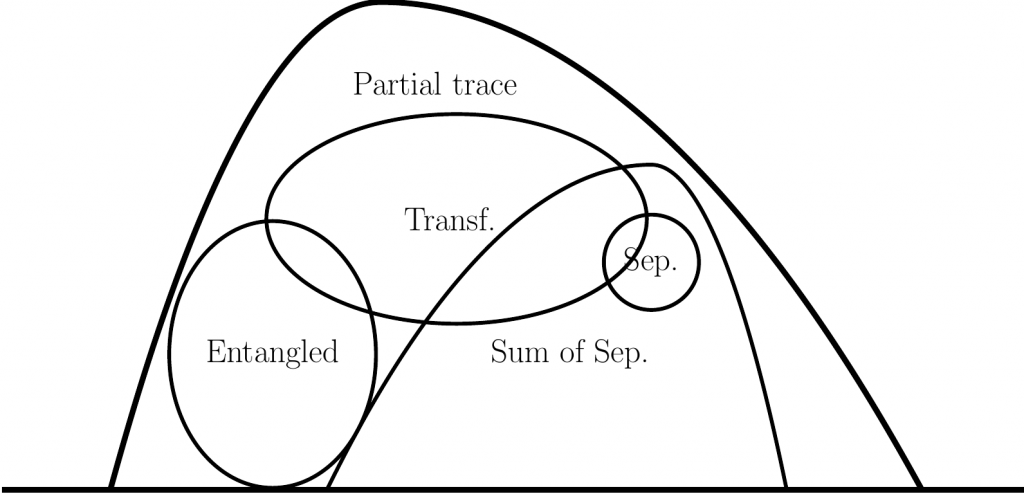
Operator-valued kernels appropriately generalize the well-known kernels used in machine learning. Especially the class of separable operator-valued kernels has been successfully applied in many learning tasks. In this work we investigate going beyond this restrictive class and derive a framework for learning non-separable operator-valued kernels. We define two new classes of operator-valued kernels, partial trace kernels and entangled kernels, and give an algorithm that learns the entangled kernels. We experimentally show the advantage of our method.
If you want to learn more about that, the full paper is available on the open archive HAL.
Riikka will make a 10 minutes talk and a poster presentation.